Synthetic Data Is a Dangerous Teacher
Synthetic Data Is a Dangerous Teacher
Synthetic data refers to artificially generated data that mimics real data, often used in machine learning and data analysis.
While…
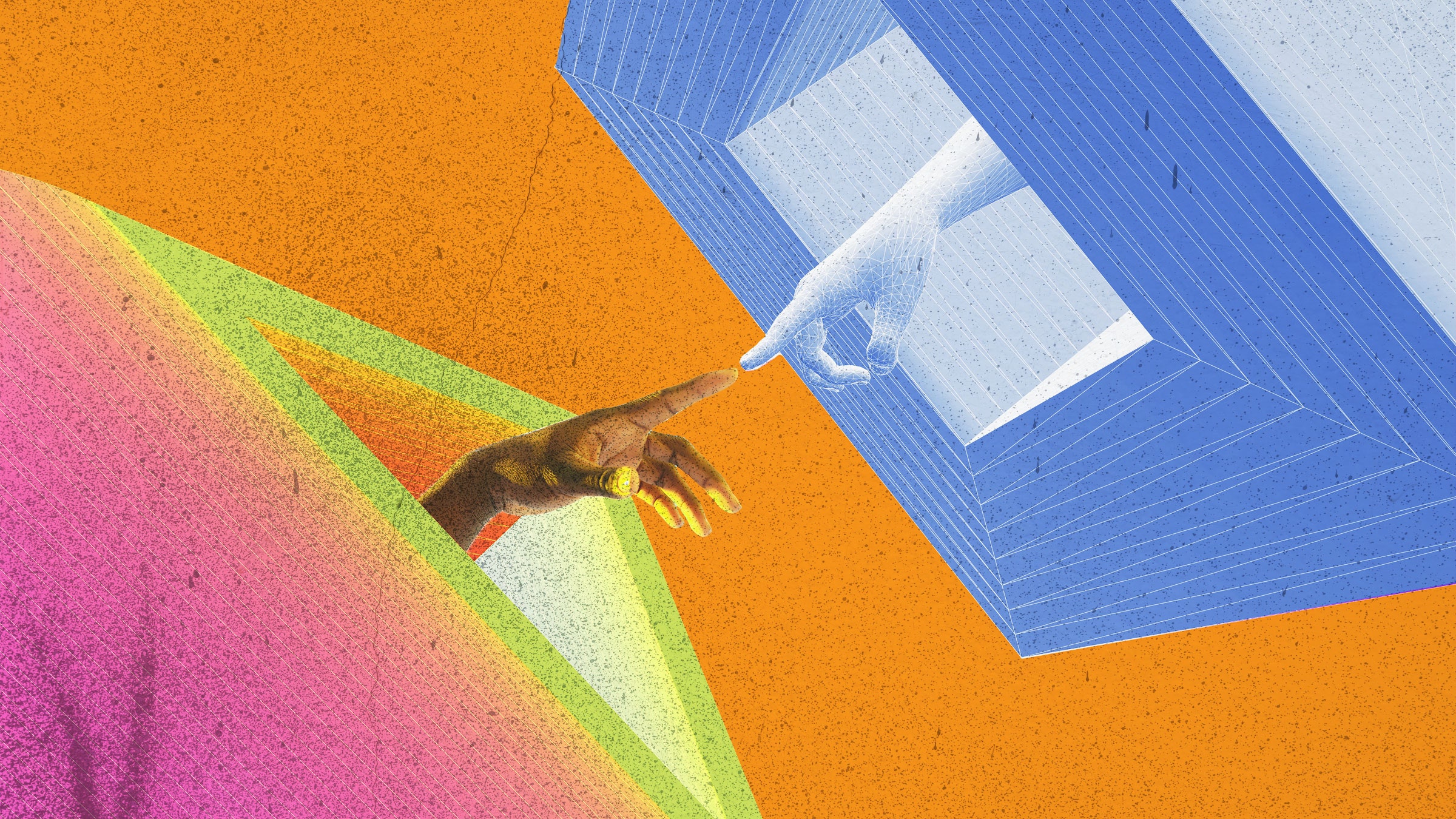
Synthetic Data Is a Dangerous Teacher
Synthetic data refers to artificially generated data that mimics real data, often used in machine learning and data analysis.
While synthetic data can be useful for testing algorithms and models, it can also be a dangerous teacher.
One of the risks of using synthetic data is that it may not accurately reflect the complexities and nuances of real-world data.
This can lead to biased or incorrect conclusions and decisions based on the synthetic data.
Furthermore, synthetic data can create a false sense of security, as models trained on synthetic data may not perform well when applied to real data.
Another danger of synthetic data is the potential for overfitting, where a model learns the noise in the synthetic data rather than the underlying patterns.
This can result in models that perform well on the synthetic data but fail to generalize to new, unseen data.
Therefore, it is crucial to approach the use of synthetic data with caution and to validate models trained on synthetic data with real data.
Ultimately, while synthetic data can be a valuable tool, it is important to recognize its limitations and potential pitfalls.